Australian organisations have tried hard to bring data together in recent decades. They have moved from data marts, which contained information specific to business units, to data warehouses, data lakes and now lakehouses, which contain structured and unstructured data.
However, the concept of the federated lakehouse could now be winning the day. Taking off in the U.S., Vinay Samuel, CEO of data analytics virtualisation firm Zetaris, tells TechRepublic reality is forcing organisations to build roads to data where it resides rather than attempt to centralise it.
Zetaris founders realised data could never be fully centralised
TR: What made you decide to start Zetaris back in 2013?
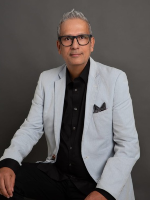
Samuel: Zetaris came out of a long journey I had been on in data warehousing — what they used to call the big database world. This is back in the 1990s, when Australian banks, telcos, retailers and governments would collect data mostly for decision support and reporting to do (business intelligence) kind of things.
PREMIUM: Key features businesses should consider when choosing a cloud data warehouse.
The one thing we learned was: Customers were continually trying to find the next best data platform. They continually started projects, attempted to join all their data, bring it together. And we asked ourselves, “Why is it that the customer could never get to what they were trying to achieve?” — which was really a single view of all their data in one place.
The answer was: It was just impossible. It was too hard to bring all the data together in the time that would make sense for the business decision that was needing to be resolved.
TR: What was your approach to solving this data centralisation problem?
Samuel: When we started the company, we said, “What if we challenge the premise that, to do analytics on data or reporting on your day-to-day, you have to bring it together?”
We said, “Let’s create a system where you didn’t have to bring data together. You could leave it in place, wherever it is, and analyse it where it was created, rather than move it into, you know, the next best data platform.”
That’s how the company started, and quite frankly, that was a huge challenge. You needed massive compute. It needed a new type of software; what we now call analytical data virtualisation software. It took us a long time to iterate on that problem and land on a model that worked and would take over from where organisations are today or were yesterday.
TR: That must seem like a great decision now AI is really taking off.
Samuel: I guess we landed on the idea fairly early in 2013, and that was a good thing because it was going to take us a good five to six or seven years to actually iterate on that idea and build the query optimizer capability that enables it.
This whole shift towards real-time analytics, towards real-time AI, or generative AI, has meant that what we do has now become critical, not just a nice to have idea that could save an organisation some money.
The last 18 months or so have been unbelievable. Today, organisations are moving towards bringing generative AI or the kind of processing we see with Chat GPT on top of their enterprise data. To do that, you absolutely need to be able to handle data everywhere all over your data lake. You don’t have the time or the luxury to bring data together to clean it, to order it and to do all the things you have to do to create a single database view of your data.
AI growth means enterprises want to access all data in real time
TR: So has the Zetaris value proposition changed over time?
Samuel: In the early years, the value proposition was predominantly about cost savings. You know, if you don’t have to move your data to a central data warehouse or move it all to a cloud data warehouse, you will save you a lot of money, right? That was our value proposition. We could save you a lot of money and enable you to do the same queries and leave the data where it is. That also has some inherent security benefits. Because if you don’t move data, it’s safer.
While we were definitely doing well with that value proposition, it wasn’t enough to get people to just jump up and say, “I absolutely need this.” With the shift to AI, no longer can you wait for the data or accept you’ll only do your analytics on the part of your dataset that’s in the data warehouse or data lake.
The expectation is: Your AI can see all your data, and it’s in a shape ready to be analysed from a data quality point of view and a governance point of view.
TR: What would you say your unique selling proposition is today?
Samuel: We enable customers to run analytics on all the data, no matter where it is, and provide them with a single point of access on the data in a way that it’s safe to do so.
It’s not just being able to provide a user with access to all the data in the cloud and across the data centre. It’s also about being cognizant of who the user is, what the use case is, and whether it’s appropriate from a privacy, governance and regulatory point of view and managing and governing that access.
SEE: Australian organisations are struggling to balance personalisation and privacy.
We have also become a data server for AI. We enable organisations to create the content store for AI applications.
There’s a thing called retrieval-augmented generation, which allows you to augment the generation of (a large language model) answer to a prompt with your private data. And to do that, you’ve got to make sure the data is ready and it’s accessible — it’s in the right format, it has the right data quality.
We are that application that prepares the data for AI.
Data readiness is a key barrier to successful AI deployments
TR: What problems are you seeing organisations having with AI?
Samuel: We’re seeing a lot of companies wanting to develop an AI capability. We find the first barrier they hit is not the challenge of getting a bunch of data scientists together or finding that amazing algorithm that can do mortgage lending or predict usage on a network, depending on the industry the customer is in.
Instead, it’s to do with data readiness and data access. Because if you want to do ChatGPT-style processing on your private data, often the enterprise data just isn’t ready. It’s not in the right shape. It’s in different places, with different levels of quality.
And so the first thing they find is they actually have a data management challenge.
TR: Are you seeing a problem with hallucinations in enterprise AI models?
Samuel: One of the reasons we exist is to negate hallucination. We apply reasoning models, and we apply various techniques and filters, to check the responses that are being given by a private LLM before they are consumed. And what that means is that it’s usually checked against the content store that’s being created from the customer’s private data.
So for instance, a simple hallucination could be that a customer in a bank, who’s in a lower wealth segment, is offered a massive loan. That could be a hallucination. That just simply won’t happen if our tech is used on top of the LLM because our tech is talking to the real data and is analysing that customer’s wealth profile and applying all the regulatory and compliance rules.
TR: Are there any other common data challenges you are seeing?
Samuel: A common challenge is blending different types of data to answer a business question.
For instance, large banks are collecting a lot of object data — pictures, sound, device data. They’re trying to work out how to use that in concert with traditional sort of transaction bank statement data.
It’s quite a challenge to work out how you bring both those structured and unstructured data types together in a way that can enhance the answer to a business question.
For example, a business question might be, “What is the right or next best wealth management product for this customer?” That’s given my understanding of similar customers over the last 20 years and all the other information I have from the internet and in my network on this customer.
The challenge of bringing structured and unstructured data together into a deep analytics question is a challenge of accessing the data in different places and in different shapes.
Customers using AI to recommend investments, heal networks
TR: Do you have examples of how you help customers make use of data and AI?
Samuel: We have been working with one large wealth management group in Australia, where we are used to write their recommendation reports. In the past, an actual wealth manager would have to spend weeks, if not months, analysing hundreds, if not thousands, of PDFs, image files, transaction data and BI reports to come up with the right portfolio recommendation.
Today, it’s happening in seconds. All of that is happening, and it’s not a pie chart or a trend, it’s a written recommendation. This is the blending of AI with automated information management.
And that’s what we do; we blend AI with automated information management to solve that problem of what’s the next best wealth management product for a customer.
In the telecommunications sector, we’re helping to automate network management. A big problem telcos have is when some part of their infrastructure fails. They have about five or six different potential reasons why a tower is failing or their devices failing.
With AI, we can quickly close in on what the problem is to enable the self-healing process of that network.
TR: What is particularly interesting in the generative AI work you are doing?
Samuel: What is really amazing for me is that, because of the way we are doing it, our technology now enables normal human beings who don’t know how to code to talk to the data. With generative AI on top of our data platform, we’re able to express queries using natural language rather than code, and that really opens up the value of the data to the business.
Traditionally, there was a technical gap between a business person and the data. If you didn’t know how to code and if you didn’t know how to write SQL really well, you couldn’t really ask the business questions you wanted to ask. You’d have to get some help. Then, there was a translation issue between the people who are trying to help and the business practitioner.
Well, that’s gone away now. A smart business practitioner, using generative AI on top of private data, now has that capability to talk directly to the data and not worry about coding. That really opens up the potential for some really interesting use cases in every industry.
Australia follows America in seeing value of federated lakehouse
TR: Zetaris was born in Australia. Are your customers all Australian?
Samuel: Over the last 18 months, we’ve had quite a strong focus on the American market, especially in the industries that are moving fastest, like healthcare, banks, telcos retailers, manufacturers, and we’re getting some government interest as well. We now have about 40 people.
Australia is the hub, but we’re spread across the Philippines and India and have a small footprint in America.
The use cases are interesting and are to do with analysing the data anywhere with generative AI. For instance, we’re now helping a large hospital group do triage. When a patient comes into the group, they are using generative AI to very quickly make decisions on whether someone’s chest pain is a panic attack or whether it’s actually a heart attack or whatever it is.
TR: Is Australia coming closer to adopting the idea of the federated lakehouse?
Samuel: The (Australian) market tends to follow the American market. It is usually about a year behind.
We see it loud and clear in America that a lakehouse doesn’t have to mean centralised; there’s an acceptance of the idea that you’ll have some of your data in the lakehouse, but then, you’ll have satellites of data anywhere else. And that’s been driven by reality, including companies having several footprints across the cloud; it’s not unusual for most enterprises to have two or three cloud vendors supporting them and a large data centre footprint.
That’s a trend in America, and we are starting to see shoots of that in Australia.
Change will not allow data consolidation in a single location
TR: So the idea of centralising organisational data is still impossible?
Samuel: The notion of bringing it together and consolidating it in one data warehouse or one cloud — I believe, and we still believe — is actually impossible.
We saw the difficulty banks, telcos, retailers and governments faced when we started with decision support and information management, and quite frankly, the mess data was and still is in large enterprises. Because data comes in different shapes, levels of quality, levels of governance and from a myriad of applications from the data centre to the cloud.
Particularly now, when you look at the speed of business and the amount of change we’re facing, applications that generate data are continually being discovered and brought into organisations. The amount of change doesn’t allow for that single consolidation of data.