Bell Canada uses AI and machine learning to improve fraud detection delays on targeted schemes by 200%
Read how Bell Canada leveraged TM Forum business assurance and AI best practices, and learnings from the TM Forum Catalyst Program, to implement a fraud-detection solution that “self-tunes” using AI and machine learning.
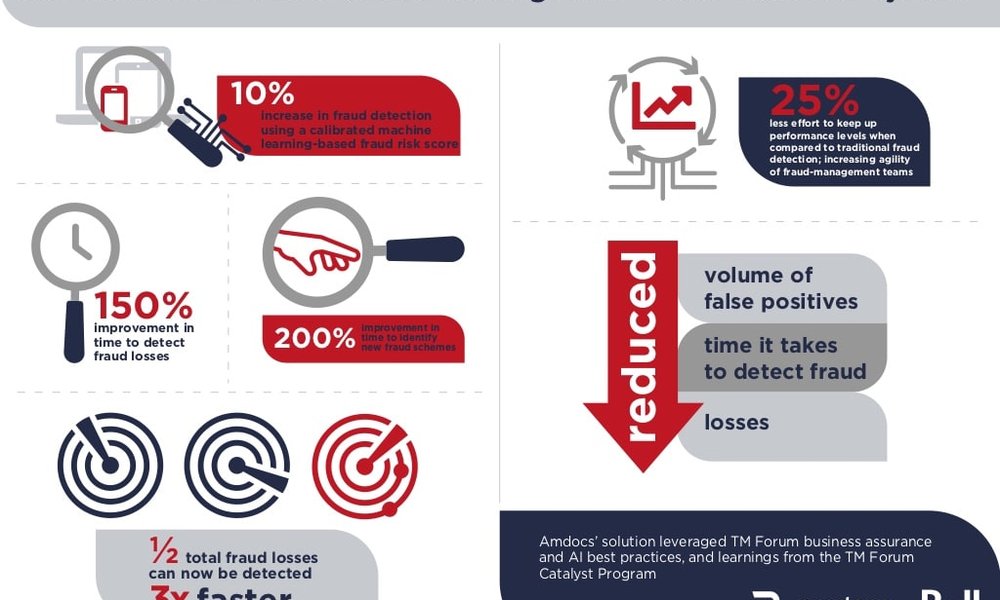
Bell Canada uses AI and machine learning to improve fraud detection delays on targeted schemes by 200%
Who:
Bell Canada and Amdocs
What:
Implemented a fraud-detection solution that “self-tunes” using AI and machine learning to improve fraud detection and reduce the time it takes to identify new schemes and losses
How:
Bell Canada’s solution was developed by Bell Canada and Amdocs, with Amdocs leveraging TM Forum business assurance and AI best practices, and learnings from the TM Forum Catalyst Program.
Results:
Bell Canada has realized a 10% increase in fraud detection, a 150% improvement in time it takes to detect losses to fraud, and a 200% improvement in the time required to identify new fraud schemes
The telecommunications industry loses close to 2% of global revenues every year to fraud. That may not sound like a lot, but considering that global telecoms revenue was more than $1.5 trillion in 2020, the losses are significant. To detect fraudulent activities, Bell Canada has partnered with Amdocs to deploy an innovative solution that uses AI and machine learning – through self-learning and auto-tuning capabilities, the model automatically adapts to learn the constantly-changing behavior of fraudsters.
The Communications Fraud Control Association (CFCA) conducts a survey every two years about fraud in the telecommunications industry. Its 2019 report found that two-thirds of respondents were experiencing an increase in fraudulent activity. The report also identified the most prevalent types of fraud which include subscription and payment fraud; PBX and IP-PBX hacking; Wangiri callback schemes; abuse of weaknesses in networks or devices; dealer fraud; subscriber and identify fraud; account takeover; and internal (employee) fraud.
“Fraud is changing,” explains Hezi Zelevski, Head of Product Management at Amdocs cVidya. “For example, fraud on devices is increasing with the proliferation of smart-home and mobile-health devices.” SIM swapping is also on the rise. This is a very concerning trend and telcos are much preoccupied by the security of their customers.
In addition to these changes, the Covid-19 pandemic has altered the behavior of both fraudsters and customers, making it difficult to distinguish between them. Customers are less likely to visit retail shops to purchase phones or make changes to their plans, so fraudsters see an opportunity to impersonate them online. Plus, the pandemic has resulted in financial losses, making some people more likely to commit fraud.
Identifying the fraudsters
Bell Canada wanted to be able to detect changes in the behavior of fraudsters much more quickly and identify customers at risk of committing fraud. Importantly, the company needed to be able to distinguish fraudsters from legitimate customers.
Bell Canada is using a solution from Amdocs that is capable of “self-tuning”, which means it uses machine learning and an AI algorithm to automatically retrain the model and adapt it to enable near real-time detection of fraudsters.
“By using machine learning, we can detect changes in usage. The system can immediately learn from it, and create models to cope,” says Dr. Gadi Solotorevsky, CTO at Amdocs cVidya, the leader of several TM Forum Business Assurance catalysts, predominantly around machine learning, and the co-head of TM Forum’s Business Assurance group. “And with AI, you find things you didn’t expect.”
For example, if the system initially identifies an account as a fraud risk, and it is later determined that they are not, the system can auto-tune the model based on what it has learned. In machine learning and AI language, this is known as a “false positive”. Similarly, the system can learn from a “false negative”.
“Our solution automatically learns and improves over time from its experience – from errors, misclassifications, and also from correct predictions,” explains Solotorevsky.
This is different from how both traditional rule-based systems and typical machine learning systems work. With a rule-based system, it is necessary to know what to look for and how to look for it, while a drawback to most machine learning systems is that they need costly manual maintenance to adapt the model.
Proving the concept
A previous project called Empowering business assurance with artificial intelligence focused on using big data analytics and AI to implement a variety of algorithms and models. The team used behavioral analysis, natural language processing and sentiment analysis for specific uses cases including identity authentication, credit checks, internal fraud prevention, prevention of provisioning failures, detecting impersonation, improving customer experience and prevention of partner fraud.
“The goal of the Catalysts was to understand what can be solved using AI, and where the boundaries are,” Solotorevsky says. “As a result, we created a common language to communicate with our partners like Bell Canada.”
Watch this video to learn more:
Significant improvement
Bell Canada has been using the AI and machine learning-based fraud detection system since November 2020. During that time, the company has realized a 10% increase in fraud detection using a calibrated machine learning-based fraud risk score. The company has also experienced a 150% improvement in the time it takes to detect fraud losses in a significant portion of its fraud, and a 200% improvement in the time required to identify new fraud schemes.
“Half of our total fraud losses can now be detected three times faster,” says Bell Canada. “Plus, we have reduced the volume of false positives, cutting the time it takes to detect fraud directly reduces our losses.”
The use of machine-learning, auto-tuning algorithms has also helped Bell Canada’s fraud-management staff. “Thanks to the Amdocs fraud detectionsolution, we achieved a 25% reduction in effort to keep up performance levels when compared to traditional fraud detection,” says Bell Canada. “This increased the agility of our fraud-management teams.”