Identifying barriers to data governance
Governance enables CSPs to mitigate risk from poor data management and extract value from the data. TM Forum's survey finds they face many governance issues
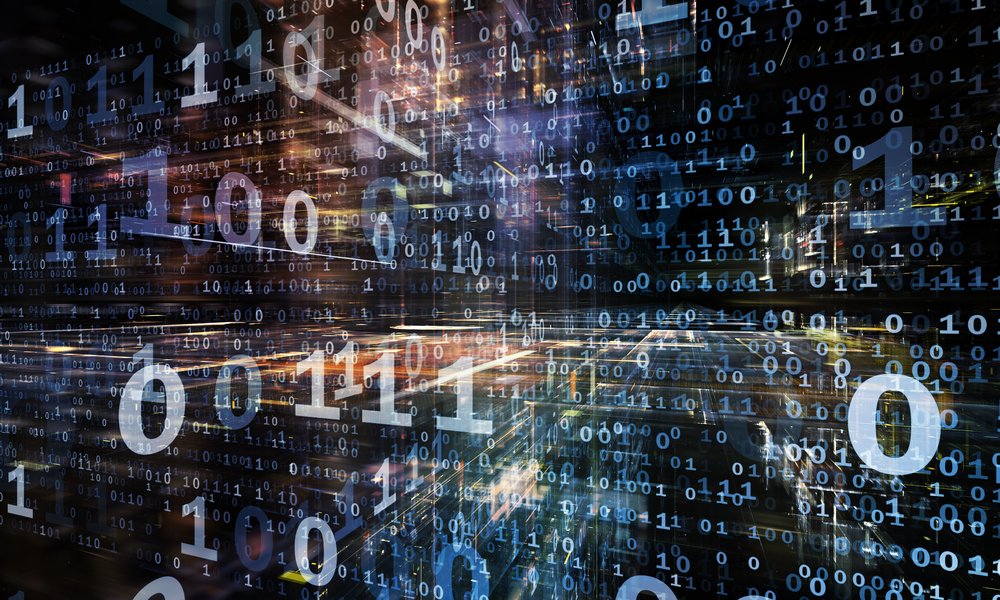
Identifying barriers to data governance
Governance enables CSPs to mitigate the business risks associated with poor data management practices while enabling them to obtain value from their data. But CSPs face many pressing data governance issues, with one of the biggest today being data silos, according to respondents to a TM Forum survey for our report The growing importance of data governance. A third or more of those surveyed also picked organizational issues such as lack of skills, insufficient leadership engagement and lack of employee compliance with policies. When we asked about the future, we found lack of agreement among respondents about the most significant challenges that lie ahead, with nearly all choices ranking medium-high. The increasing number of uses for realtime data scored slightly higher, pushed forward by the use of more automation and development of IoT services, particularly ultra-low latency services which are necessary in sectors such as automotive and healthcare.
Effect of Covid-19
We also asked a free form question about whether the pandemic has raised any new governance issues. Half of survey respondents said they have seen no new issues as a result, but for CSP respondents citing problems, the most prevalent are around the monetization of data. The requirements from government bodies to provide data on the movement of people seems to have highlighted the need for new governance around sharing of data with customers and partners in an ecosystem. Additional challenges that CSP respondents named because of the pandemic include the increase in remote working, which causes issues related to governance of data that is used off-site, and increased use of digital channels and self-service, where improved data quality is needed for creating personalized online experiences.
Maturity matters
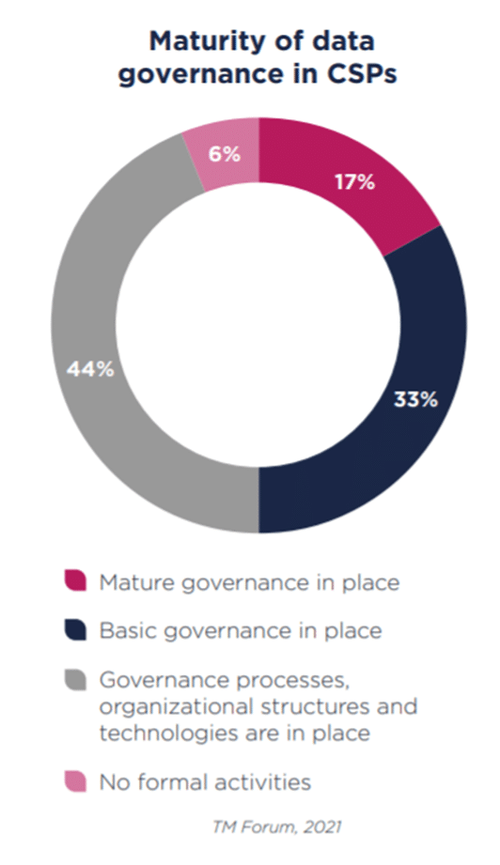
With issues such as data silos and organizational challenges ranking high on the list of challenges for many CSPs, they are clearly still in the middle of building maturity in their governance programs. This can be seen in the response to a question about maturity, which shows that only 17% of respondents consider their companies to have a mature governance program in place and 6% still have not even started the journey. In the later stages of maturity, an organization will achieve goals in areas such as breadth of data covered, data quality and employee compliance. It will also have metrics to measure the value created with reference to corporate focus areas. For example, if the CSP is investing time and resources in building a position within a particular IoT ecosystem, metrics will need to measure the degree of successful governance between the CSP and all its partners. A mature CSP will also have governance goals that look to the future and ensure that models are agile enough to support market and corporate change.
- Policies and standards that are deployed and understood in the organization
- A centralized governance body that can think strategically about the governance needs of the organization and includes specialists who can focus on tasks such as compliance and data protection
- Basic metrics related to governance processes (for example, How long does it take to solve data-related errors? What governance dashboards are available?)
- An understanding that information is a critical asset
Focus on the future
As noted, our survey shows that consensus is lacking around the most pressing future issues. Following is our analysis of higher scoring issues:
Real-time data – IoT increasingly will provide new data management challenges for CSPs because data will flow through a constantly reconfiguring mesh of connected components. Some IoT solutions such as connected cars will be mission critical, directly affecting the wellbeing of the population. This data is likely to present interesting governance issues as it is generated by multiple, non-standard devices which will each have new governance requirements.
Governance needs for AI and machine learning – as CSPs roll out more AI and machine learning, they must focus on data governance because any weaknesses in the model will be highlighted quickly as the technologies are deployed at scale. Ethical governance will take up considerable management time sparking questions for CSPs to answer (for example, How can human flourishing be put front and center of the governance policy? How can data and models be fair, reliable, safe and responsible?).
Diversity of data types – cross-vertical estimates suggest that 80% of corporate data will be unstructured in the future due to increasing amounts of video, audio, web content, and external data sources such as weather data and data taken from social media. Part of the solution is the deployment of management systems which “structure” such data – for example, customer relationship management records or Sharepoint solutions. But it is assumed from the importance in our survey that there is still need for an expansion of the governance process to monitor a broad portfolio of data stores, catalog key metadata, classify sensitive data, map users, define authorization policies, provide metrics and quantify the extent of any vulnerabilities.
New governance around the cloud – although CSPs are in the process of moving data to the cloud, this was not the most pressing issue identified. Even though cloud platforms require multiplication of capabilities to manage user access permissions, governance, auditing and data lineage (understanding who is using data, what information it contains and when it was created/transformed), CSPs are finding that they can extend existing systems or use integrated solutions from the hyperscale platform providers.
New governance needs for humans and machines – considering a workplace where employees work alongside AI and machine learning, early AI implementations are likely to find machines surfacing internal insight from existing data and providing it to a human, while future capabilities will look externally and bring insight from secondhand third-party data via searches and data requests. Intelligence can either make simple suggestions and fulfill simple tasks for the human, or undertake more complex decision-making for knowledge workers in the organization. Data governance requirements remain unclear, but machines will have to be considered data consumers with their own access permissions. Governance maturity = policies, people, tools & commitment Cross-vertical estimates suggest that 80% of corporate data will be unstructured in the future due to increasing amounts of video, audio, web content, and external data sources such as weather data and data taken from social media.
Increasing quantities of data – big data storage capabilities have weakened the link between the collection of data and the pre-defined purpose for that collection. With larger volumes now collected and analyzed in ways that generate unexpected insights, the collection can go far beyond the original intention. This issue will be exaggerated by the use of composable analytics (which use lowcode or no-code capabilities to create new applications from already existing analytics assets).
Data monetization, platform business models and peer-to-peer initiatives – platform models, open data and peer-to-peer working will require data to be passed between parties necessitating governance. Policies need to be understood by all parties, and there will be additional rules around anonymization. CSPs also will need to work on policies for permission management to ensure clarity around what third-party partners can do with the data which is potentially more likely to move between countries.
Download the report The growing importance of data governance.