‘Algos’ vs. autonomy: What CSPs can learn from financial markets
Many in the communications sector marvel at the meteoric rise of AI and general automated software in the financial markets. Certainly, the sector has moved faster than most. But there is a difference between automation and autonomy.
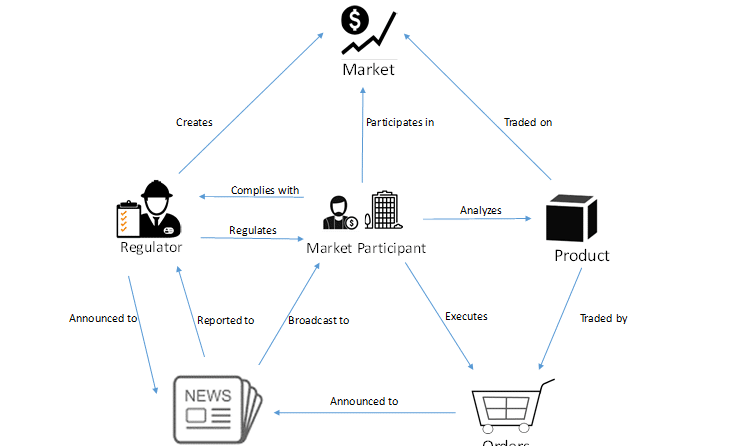
‘Algos’ vs. autonomy: What CSPs can learn from financial markets
Many people in the communications sector marvel at the meteoric rise of AI (and automated software in general) in the financial markets. Certainly, the sector has moved faster than most. But there is a difference between automation and autonomy. A lot of hype exists around the term “algos”, short for algorithms. Within the financial markets ecosystem – which includes the market itself, market participants (buyers and sellers), products, regulators and news sources about the market (depicted below) – the market participant is the main role that has become most AI or algo powered. This role can be an institution or an individual.
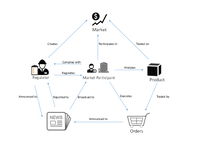
In the vast majority of cases, however, these algorithms, while automated, are not highly autonomous.
Trading vs. algorithmic trading
The economic objective of trading for the majority of public financial market participants can be roughly summarized as: Buy low, sell high – and do it before anyone else! So why don’t we all just do that, then? Many of us do, in theory, but the only market participants that maximize their economic objective functions are: The majority of investment around “algorithmic trading” software focuses on these optimization problems. Things change extremely quickly in the markets, triggered by all types of news and events. Having a competitive edge in this business is challenging, as everyone has access to the same market data. Consequently, many companies use “outside” data sources to get ahead. For example, they may use satellite images to track the number of cars in the parking lots of retailers like Walmart, Target, Home Depot or Tesco to infer trading activity and predict future stock prices. Or they may use such images to track the volume of daily crude oil inventory stored in floating roof tanks to predict future crude prices.
- Those with product knowledge advantages (i.e. they can effectively determine what is “high” and “low”)
- Those that have an order-execution advantage (being first matters)
Many of the algorithms that are used are “disposable” – what worked today will not work tomorrow. Plus, trading strategies need to constantly evolve, and software engineers (or quantitative analysts with programming skills) are constantly rewriting and back-testing algorithms. There is a lot of automation, but the much hyped “algo” is not an autonomous silver bullet.
Autonomous wealth management
Wealth management, on the other hand, seem like a boring topic, but is where the action is when it comes to autonomous behavior. Robo-advisors are digital platforms that provide automated, algorithm-driven financial planning services with little or no human supervision. The graphic below illustrates a typical customer journey for an investor using a robo-advisor.
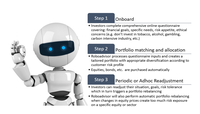
The best robo-advisors put customer experience first, offering easy account setup, robust goal planning, account services, portfolio management, security features, attentive customer service, and – importantly – low fees. There are about 100 robo-advisors in Europe alone, and the market is growing quickly. Betterment, Nutmeg, and Moneyfarm are examples of some popular robo-advisors. On trust and perceived risk of robo-advisors, it’s important to acknowledge that internet and mobile banking has really paved the way for the trust and adoption of robo-advisors amongst consumers, especially with the younger demographic (“digital natives”).
In general people do not view it as riskier than giving their money to a traditional financial company to invest on their behalf. Notably, across all age demographics robo-advisors that provide some hybrid engagement (i.e. human and computer) options are more trusted than those that do not, even though the fees are higher. For finance practitioners trust has been fostered by providing override and control options to humans.
An inspiration and template for CSPs
Robo-advisors have democratized the investment world by making investing accessible and extremely simple. Customers need absolutely no knowledge of the financial markets to get involved. For CSPs that want to grow their businesses through offering services to enterprises and vertical industries, the robo-advisor concept is a good template of what is needed: Customers specify their intent and the robo-advisor does the rest. Customers can see the real-time status of their account at any stage and modify intent as required, at which point the robo-advisor will reconfigure things accordingly. For CSPs to be credible ICT providers across multiple industries and sectors, they need to deliver services with a similar level of consumability and simplicity for customers. None of the networking complexity should be exposed to the customer, and the experience should be zero-touch, zero-wait, zero-trouble.
The autonomous behavior of robo-advisors is not just at the customer engagement level. Internal processes are also autonomous to optimize the buying and selling power of multiple customers’ investments, to subsequently optimize returns made at the aggregate level.
The autonomous capabilities make the business lower cost to operate, but it’s important to stress that there are still humans involved to monitor the process of the autonomous agents. Through the application of machine intelligence, however, human tasks and decision making are simplified with explainable situation summaries and action suggestions. Chatbots are the normal customer care option, but human contact is also still an option (though most of the demographic using robo-advisors prefer chatbots).
Takeaways and recommendations for CSPs
- The robo-advisor is an excellent conceptual business model target for how CSPs should support enterprise and industry verticals. The concept works because it focuses on providing a simple, frictionless customer journey. Autonomous networking principles centered around intent and closed control loops can help them achieve this target. To help promote trust and adoption some human customer touchpoints may still be worthwhile to complement the autonomous services however.
- Financial companies regularly look outside sector-specific data sources to gain competitive advantage. As they look to penetrate further into vertical industries, CSPs should consider what is appropriate for them in this regard.
- Information aggregators within the finance sector have focused on human-readable and decision-ready APIs. CSPs and the telco industry should incorporate decision-ready principles into API design to make interoperability easier and to accelerate autonomous behavior at higher-stack layers – this will become more important as customers increasingly come from outside the telco domain.
- Volatility is a critical metric in investing, but it is not used directly in the telco sector, despite the very heavy reliance on statistics. Given the increased dynamicity in networking and use of AI, volatility could be worth considering to enhance decision making within operation and maintenance. That is: consider the upside (or downside) volatility of a service resource metric along with the actual metric itself.
A more detailed analysis is available as a contribution within the TM Forum Autonomous Network Project. The technical architecture elaboration currently being worked on by the team is largely focused on intent and closed loop automation design, with the aim of bringing capabilities to networks similar(in concept) to that of a robo-advisor. The first technical architecture output is planned for October 2020.
To find out how you can join the Autonomous Networks Project, please contact Ian Turkington, VP of Architecture & APIs, TM Forum. You can also learn more about the group by reading these related project outputs and articles:
TMF Autonomous Networks White Paper
IG1218 Autonomous Networks business requirements and architecture v1.0