Maximizing AI ROI Through Change Management
To make AI’s promising impact a reality, data science teams must see themselves as change agents and treat their work as mission-critical business matters.
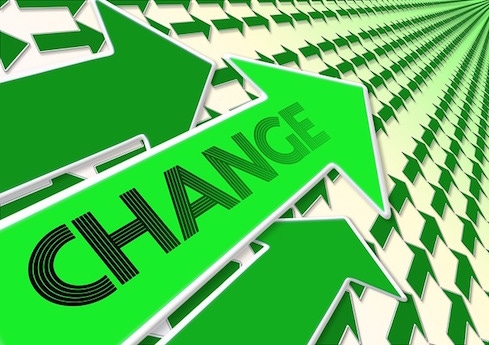
Thanks to ChatGPT and the rise of large language models, artificial intelligence seems to have entered another of disproportionate fanfare and hope. While people are placing new bets on the latest developments in AI, speculation and doubt still lurk in the shadows -- “Can this technology really change the game for me?” and “Is it different this time around?”
These doubts are well justified. Those in the data industry have seen no shortage of statistics that look like this:
Only 10% of companiesobtain significant financial benefits from AI
5 in 6 machine learning models do not go into production
77% of businessesreport that big data and AI adoption is a challenge
Despite the potential, many companies have struggled to effectively implement AI, hampering their ability to reap the benefits of their investments. Even with decent data and tools, compelling use cases and leadership mandates, too many AI and ML projects are still at risk of failure. This begs the question: Will the new generation of AI and ML technologies face the same fate?
The key ingredient for success has been and will always be the same: change management.
There’s a misconception that “achieving” ML is a technical matter. This may be the case for digital-native companies that are inherently built on data-driven products and processes. But for most companies trying to embed AI and ML from the outside in, advanced concepts such as metadata logging, CI/CD automation, and versioning are tangential compared to the outcome they really need: adoption.
Over-indexing on technical concepts is what causes well-intentioned, skilled data science teams to only develop proof-of-concept models and then enter into a state of pilotitis -- the pattern of pursuing small projects but never scaling them. The tables must turn. Businesses must make the subtle, but powerful paradigm shift that AI is an absolute business matter. This is the prerequisite to the exploitation of AI and ML for traditional enterprises.
Change Management Paves the Way to ROI
Business teams do not always fully trust data. What if we lose 15% in sales revenue with the new pricing model? What if a bad actor slips through the automated loan approval process? This is understandable; the stakes are high. By implementing ML in their domain, business stakeholders may be seen as rocking the boat, or feel they are putting their reputation or careers on the line. That’s where data science teams come in. They need to earn stakeholder trust, focusing on business, risk, finance, and legal partners. This process is more fundamental than any operating model.
Whether it’s a handful or hundreds of impacted users, change management frameworks such as Prosci’s Awareness, Desire, Knowledge, Ability, and Reinforcement (ADKAR) framework can be employed. In this framework, the individual components need to happen sequentially. We will use ADKAR as an example, but any change management framework can be applied. Here’s what it looks like:
Awareness: Stakeholders need a baseline awareness and to acknowledge that a problem or opportunity exists. They should also understand there’s a risk of not changing, whether it’s competitive pressure or increased regulatory scrutiny.
Desire: Despite understanding the issue, people don’t always have the impetus to change. Data science teams need to help business leaders translate the manifestations of the ML solution to communicate the expected benefits.
Knowledge: All parties need to know what exactly needs to be done differently to adopt the solution. Data experts need to educate business teams about the data, algorithm, and governance limitations and co-define how to interact with the ML system.
Ability: Business users must apply the new ways of working and demonstrate their ability to generate the desired results. The ML solution should be rolled out incrementally because glitches and opportunities for quick remediation are inevitable. Clear roles, triage and support should be established for issues that occur, and all feedback clearly documented.
Reinforcement: This final step is crucial to ensuring changes stick. Data science teams should leverage model feedback loops and systemically log and analyze the results of how the ML system manifested into a business process. ML adoption rates ought to be shared in regular forums; where there are adoption gaps, leaders should ideate new ways to support the users and ensure the right incentives are in place. This stage is also an opportunity to “seal the deal” by sharing success stories.
Technical Teams as Agents of Change
To make AI’s promising impact a reality, data science teams must see themselves as change agents and treat their work as mission-critical business matters. Gaining trust and commitment from their business partners matters more than a pristine or “production-ready” technical solution.
Data science teams have a tremendous role to play throughout the model development lifecycle to create a sense of co-ownership. For their business partners, desire to learn the business process, and problem-solving and collaboration skills cannot be understated.
Implementing AI and ML involves far more than the typical idea of MLOps alone. When technical teams see beyond data sets and algorithms and gain the trust of their business counterparts, they can make contributions that significantly move the needle for AI and ML democratization within their organizations and beyond.
About the Author(s)
You May Also Like