Tips to Achieve Enterprise ML Success
Operationalizing an ML ecosystem can help make business data a predictive engine for the business. Learn how to achieve enterprise ML success from Capital One.
October 24, 2022
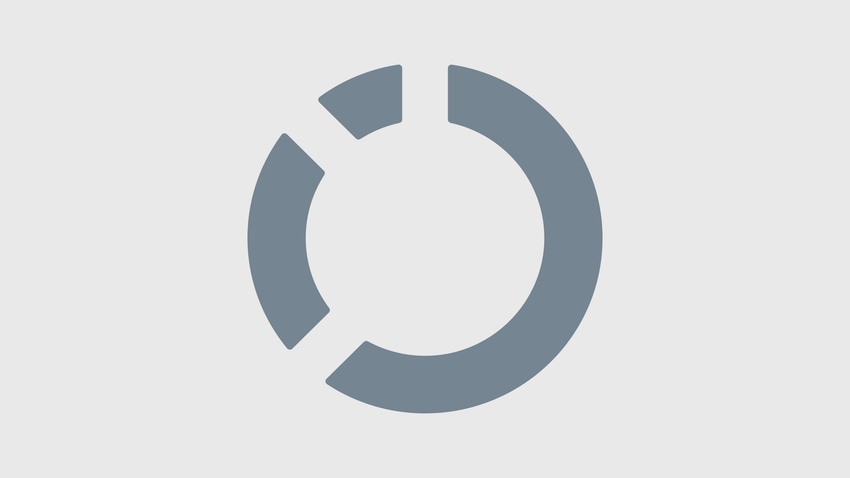
Sponsored by Capital One
(SPONSORED ARTICLE)
Data and analytics executives have always known in broad strokes the business value they can achieve from adopting machine learning (ML). The value tends to come in three ways: improving the user experience (customers and employees), generating operating efficiencies, or driving top-line growth.
But line-of-business teams face persistent challenges on the road to unleashing that value, with the number one roadblock being the inability to gain insights from their massive treasure troves of data. According to a recent data management Forrester Consulting study commissioned by Capital One, eight out of 10 data management executives cite poor data quality as their top ecosystem challenge. Other top challenges include difficulty understanding data (76%) and a lack of data observability (74%).
A new Forrester Consulting study commissioned by Capital One about operationalizing ML uncovered the root causes of organizations’ data challenges. They include difficulty translating academic models into operationalized approaches, data silos across the organization, and AI risk. Getting ML models into production is still a messy endeavor, which is why we aren’t seeing applications of ML blossom faster. More than half of the respondents in the Forrester ML study reported that their organizations had only been developing and releasing ML applications for one to two years. Many remain in the experimental phase.
But what we often see as organizations’ ML ecosystems mature, is a shift in how they measure success. They transition from seeking IT-heavy gains to seeking business decision-maker outcomes such as better digital experiences and revenue growth. The Forrester data bears this out. Data and analytics executives say their top priority right now is successfully using a multi-cloud environment. However, over the next three years, the highest priorities shift to deploying ML to automate anomaly detection.
To achieve this, democratizing ML for anomaly detection, changepoint detection, and root cause analysis is key to unlocking insights across wide-ranging use cases. For example, our open source Data Profiler solution provides a pre-trained deep learning model to monitor big data and detect private customer information so it can be protected.
Engaging business analysts more deeply in ML development and data insights was a crucial decision at Capital One and went a long way toward removing the silos between analysts, data scientists, and engineers. I wrote earlier this year in InformationWeek about how to democratize ML across the enterprise. Here I want to share some best practices in operationalizing your ML practice as a mature program:
Identify a partner. Roughly a third of ML decision-makers are working with data and platform partners (internal and external) and expect to grow that relationship. It’s always best to find a partner that has been “in the ML trenches” and proven the ability to operationalize ML apps with transparency and explainability.
Build the business case for organizational support. Decision-makers want to see ML’s positive impact across the organization, so it’s always best to build a business case that delivers cross-business outcomes. Some benefits to focus on include easier data mobility, traceability, and faster time-to-action. Once you establish the proof points around better CX and revenue growth and put some verified wins on the board, it becomes much easier to keep leadership motivated.
Standardize across teams. A best practice is to leverage a platform that provides your teams with governed access to algorithms, components, and infrastructure for reuse. This allows non-data science and machine learning practitioners to tap ML for business decisions with impactful results. An example is our use case for credit card fraud defense, where we’re using home-grown and open-source ML algorithms hosted by a shared platform to detect anomalies and automatically create defenses.
Leverage platforms for model operationalization. Custom ML model pipelines can be inefficient and unreliable, putting ML out of reach for non-expert practitioners. Standardizing on the same stack and reusing frameworks across all ML efforts using cloud-native platforms like Kubernetes helps ensure that parameters and outcomes are repeatable and searchable. Repeatability shores up your model audits and governance reviews, as well.
Most organizations are still in the late stages of the experimental phase with ML and looking for the right path toward maturity. Thinking about operationalizing an ML ecosystem is essential to reaching that higher level where business data becomes a predictive engine for your business and a fertile source of new revenue streams and business opportunities.
Dave Kang is SVP and Head of Capital One Data Insights leading an organization of data scientists, software and ML engineers as they build solutions to democratize machine learning.
You May Also Like