Enabling Edge AI To Be Truly Transformative
The adoption of edge AI is on the rise, but if it is to proliferate, there are numerous technical and logistical hurdles that will need to be overcome.
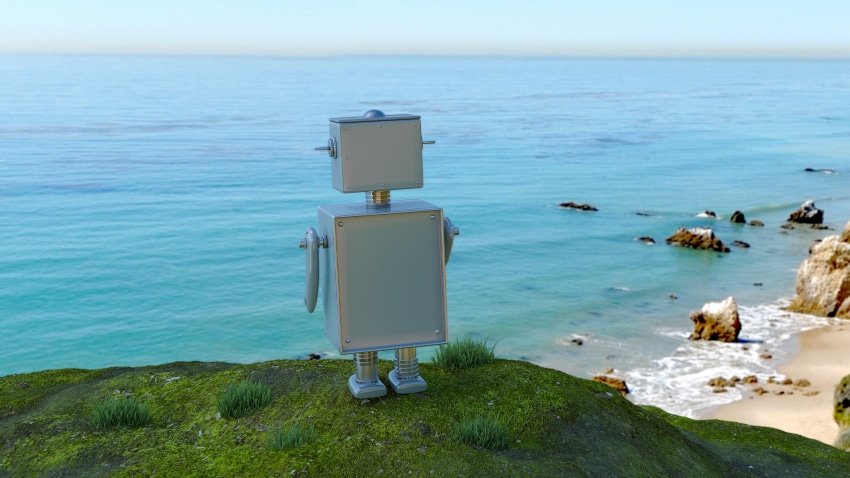
The adoption of edge artificial intelligence is on the rise across numerous industries. As this trend continues, it will bring about transformative changes not only for businesses but also for society.
The decentralized approach of edge computing mitigates constraints such as data congestion, connectivity faults and transference costs. This greatly improves the performance of AI applications, allowing for quicker, more dependable decision making.
Edge AI’s advantages are logistical as well as technical. In difficult-to-reach locations, such as oil rigs, edge AI can identify patterns that indicate heightened risk and react accordingly to prevent potentially hazardous situations. In agriculture, farmers will be able to maximize yields by enabling machinery to make autonomous decisions based on environmental conditions.
Society also stands to gain. Imagine a drone swarm capable of conducting search and rescue operations over rugged mountainous terrain, using multiple sensors to detect shapes, sounds, heat or movement, recognizing signs of life. At our company, for example, we have been deeply involved in developing edge AI models that can monitor human motion to detect signs of fatigue or injury.
As adoption of edge computing grows, so too will the applications of AI. But if edge AI is to flourish, there are numerous technical hurdles that need to be overcome.
Barriers at the Edge
Perhaps the most limiting factor for edge AI comes from the fact that edge devices are typically small, with limited computational capabilities. Their performance is a far cry from those found in data centers or even in powerful desktop GPUs. With special AI techniques such as model downsizing and quantizing, however, limited models fitting small devices can provide a lot of useful functionality.
Other challenges arise from the fact that edge computing projects often operate in hard to reach or remote locations. Providing power and connectivity to such devices can be difficult, and meeting IoT standards to ensure that these devices can talk to one another is not always straightforward.
A third obstacle is that while edge AI itself has limited reliance on the internet, many personal edge devices, such as wearables, will want to support applications that require some cloud connectivity, introducing an element of risk.
These obstacles are not insurmountable, however. A range of power and connectivity solutions can help, including long-life batteries, 5G connectivity and low power hardware architecture.
The AI Chip Challenge
The major roadblock in front of edge AI projects is the cost, performance, and power requirements of AI chips. In certain industrial scenarios, the number of IoT devices involved could extend the chip requirements to the hundreds of thousands, driving project costs sky high.
Deployment on this scale demands a meticulous evaluation of the cost-to-performance ratio, and at current prices this could prove prohibitive. Until we see a significant improvement of these AI computing factors, we are likely to see only small-scale models with limited problem-solving capabilities.
Enabling an Educated Edge
Another significant challenge is finding a way to train all these autonomous AI-enabled devices. Recent developments in generative AI (GAI) show systems such as GPT being trained on extremely large data sets available on the internet. This requires substantial effort to collect and process the data. For educated decision making at the edge, we will need to address the need for an adequate quantity of data.
If we look again at recent developments in GAI, however, the solution may have already revealed itself. One approach may be to use the ability of the generative models to produce large amounts of synthetic training data, based on a few examples provided -- and then use this data to train smaller models more quickly. Another approach, perhaps further down the line, is to train a large generative model directly on live training data (if available), and then use this to train a smaller, edge AI model.
This approach has already yielded results as seen with Orca's 13B, a much smaller model that has been able to learn from larger foundation models, such as GPT-4 -- and is producing remarkably similar outcomes. Many observers of recent AI developments claim we are on the brink of a “Cambrian explosion” of small, purpose-built AI models. These could be embedded in edge devices to provide exceptional abilities for specific tasks.
Machine-to-Machine Learning
Another avenue to faster learning involves managing an interconnected, self-improving fleet of AI-enabled edge devices from a centralized system. In many cases a feasible solution will be to have models that can be incrementally trained while being “on assignment”, and which can share important discoveries.
Similar to sharing best practices across a business or industry, machines can help to identify patterns that guide behavior.
The notion of a fleet of autonomous machines controlled by an intelligent central entity might resemble a dystopian science fiction story. So, as with anything involving AI, behavioral parameters will need to be imposed.
In the not-too-distant future it is entirely plausible that automated edge devices will have the ability to learn from each other. This will give them the capacity to make increasingly educated decisions on our behalf, which will have a transformational impact on industry and society alike.
About the Author(s)
You May Also Like
How to Amplify DevOps with DevSecOps
May 22, 2024Generative AI: Use Cases and Risks in 2024
May 29, 2024Smart Service Management
June 4, 2024