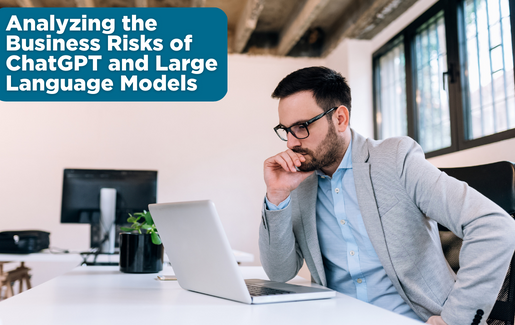
ChatGPT is a large language model (LLM) that has inspired many people because it is able to succinctly demonstrate to everyone, irrespective of role, the power of the conversational interface. Open AI, the group responsible for ChatGPT, has been upfront with its limitations, including warning labels about bias and unintended uses. ChatGPT details that its training data was curated in 2021 for example, so its knowledge of current events are limited. With all that said, the realization of what artificial intelligence (AI) is now doing to transform the world is becoming clearer and clearer—for better or for worse.
There are many unique risks that are presented from LLMs. Transparency is critical to enabling researchers and the entire downstream supply chain of users to investigate and understand the models’ vulnerabilities and biases. Developers of the models have themselves acknowledged this need.
Related blog: 4 Cybersecurity Risks Related to ChatGPT and AI-powered Chatbots
How are LLMs (like ChatGPT) being used? Here are a few common enterprise use cases, and some benefits:
- Content generation
- Automatically generate responses to customer analytics
- Generate personalized UI for websites
- Summarization
- Summary of customer support conversation logs
- SME document summarization
- Social media trend summarization
- Code generation
- Semantic search
Some Questions to Ask Early in the Process
When thinking about a framework for responsible use, it is useful to consider the various directions where risk can be introduced. There are many frameworks including human rights articles that have been published that describe potential individual and societal harm from various forms of automation. These include:
- Discrimination, exclusion and toxicity
- Information hazards
- Misinformation harms
- Human-computer interaction harms
- Automation, access and environmental harms
As managed service providers (MSPs) and other tech companies start to investigate how and when to use ChatGPT and other LLMs, there are a number of questions to consider. Some typical client questions that we have heard on the subject of LLMs include:
What are the appropriate use cases for LLMs?
Specifically, use cases that offer low-risk damage for my company, plus low risk of harming users and low risk of questionable data sourcing for my training model?
How do I know that the training data used to train my model is safe?
Has it been audited for discrimination, exclusion, toxicity, information hazards, misinformation harms, HCI harms, automation, access and environmental harm? Is it able to handle negation? Is it not inferring private information, etc. to build upon?
How are we monitoring the environmental impact of these models?
In use cases where clients need to have data lineage and provenance of the recommendations being made/information being surfaced, how do the LLMs provide that?
Who is responsible for misbehaviors and copyright issues for content produced by LLMs?
What happens if that content is challenged by a content owner?
Who is liable for content generated by LLMs?
Especially if it represents data from training data sets directly.
Hallucinations and Other Risks
It is critically important to recognize that when LLMs are asked about something they will not always give a consistent response, so the type of test and retest reliability can never be met. It is also always possible for the LLMs to render a response stitched together from multiple sources and be inaccurate.
For example, it could say that a professor from University A wrote a document when the professor is really from University B. This is exceptionally dangerous because new false knowledge can so easily and unintendedly be created. These are commonly referred to as “hallucinations.” The LLMs predict the next best syntactically correct answer, not accurate answers based on understanding of what the human is asking for.
Here's an example:
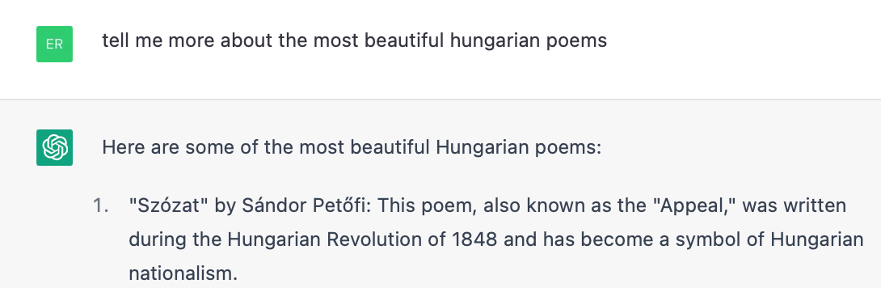
Looks like a great answer, doesn't it? But that poem was not written by that poet, demonstrating the danger of solely relying on LLMs.
Here’s another example:
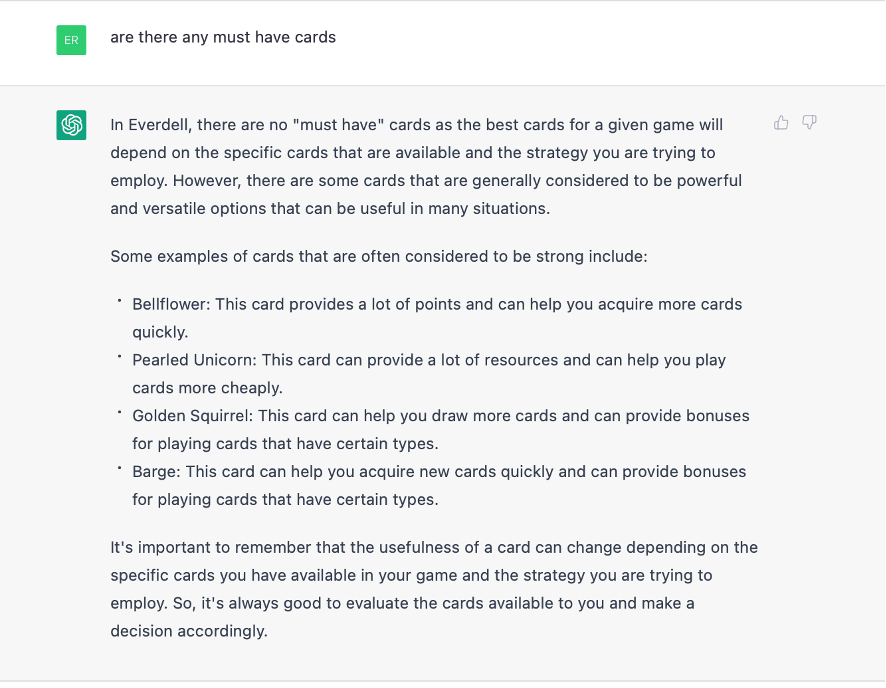
Anyone play Everdell? These cards do not exist in Everdell. More falsification, more hallucination.
It should not be assumed that a summary or classification done by AI, like a LLM, is fairer that if done by a biased human. ChatGPT, along with other LLMs, in their current form are not auditable for bias and do not have adaptable, corrective feedback loops.
If someone were to find a bias in the way an LLM was summarizing or classifying information, does the client have established processes in place to address these? Do they have guidelines, communication, education and a process to ensure that the risk of causing harm is mitigated immediately? Who is carrying out this audit, with what skill and how often, against which risk factors, etc.? Is this even the right use for AI for your business and is it aligned to business strategy?
Education, Standardization Will Be Critical
One way to achieve mass adoption of enterprise use cases of LLMs – and minimize clients’ risks and concerns about those creating content from LLMs – is to create external accreditation where practitioners must apply their skills to problems in experience-based projects using multi-disciplinary teams that are then peer-reviewed. The industry could use the example of electricians as a guide.
In 1893, at the Chicago World Fair, electricity was introduced with all its marvels to the public. What is little known is that a fire broke out due to improper wiring and killed 16 people. This tragic incident served as a catalyst for the formation of a national program to certify electricians based on agreed-to standards. Similarly, in the context of AI, we too have had our fires and recognize the need for standards and potentially certification programs in AI.
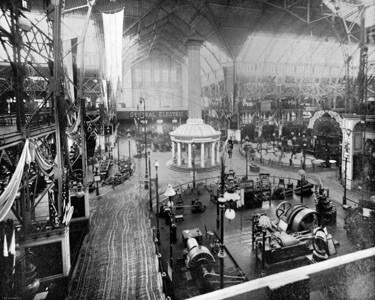
Certified data scientists have to understand business problems and how to transform them into hypotheses and do this in a supportive process complete with mentors. In order to maintain this credential, we recommend that they should have to re-certify every three years.
In conclusion, earning trust in AI is not a technical problem with a technical solution. It is a socio-technical problem that requires a holistic approach. There is nothing easy about developing AI responsibly. Take the time to consider, what is the kind of relationship that we ultimately want to have with artificial intelligence?
As doctors have the Hippocratic Oath, our AI practitioners should also have a similar oath to follow. Lori Sherer from Bain & Company suggested some of the points below which members of IBM’s Trustworthy AI Center of Excellence added to:
- I recognize that data science has material consequences for individuals and society, so no matter what project or role I pursue, I will use my skills for their well-being.
- I will take all reasonable precautions to protect the integrity of my models.
- I will always try my best to tell factual from non-factual information in my data sets and know the impact of mixing the two in a model that when used will not be able to tell fact from fiction.
- I will consider the privacy, dignity and fair treatment of individuals when selecting the data permissible in a given application, putting those considerations above the model’s performance.
- I have a responsibility to bring data transparency, accuracy and access to consumers, including making them aware of how their personal data is being used.
- I will act deliberately to ensure the security of data and promote clear processes and accountability for security in my organization.
- I will invest my time and promote the use of resources in my organization to monitor and test data models for any unintended social harm that the modeling may cause.
Companies that are dedicated to operationalizing the oath above will be in a good position to successfully differentiate themselves in this market.
Six Tips to Get You Started Operationalizing LLMs
If you’re thinking about operationalizing LLMs and AI, now’s the time to start preparing your business. Learn the models, make the investments, find the right partners so that you can have conversations with your clients and be able to answer their questions.
Here are six tips to help you get started:
- Make sure any investments made in AI tie directly to the business need and strategy. Many times we have found that AI projects get stalled in proof of concept.
- Consider the need for data lineage and provenance. Empower your users by demonstrating why the model said what it said (in-line explainability) and give them the opportunity to offer feedback that can be acted upon.
- Offer education and communication on how/when to use these technologies.
- Assess and audit pre- and post-model deployment.
- Put organizational AI governance in place in order to mitigate any risks as they arise.
- Catalog the skills of your team to determine which personnel should do what role (for example, content grounding domain expert, auditing for bias patterns, etc., post deployment), and change management support.
Want to learn more about AI?
Join a CompTIA Community in your region and participate in our Emerging Technology Committees.